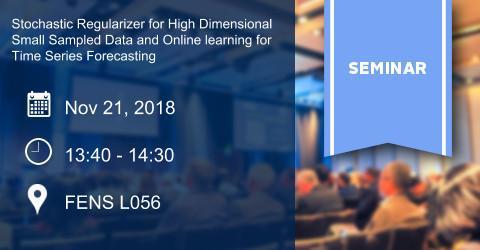
Speaker: Sergül Aydöre
Title: Stochastic Regularizer for High Dimensional Small Sampled Data and Online learning for Time Series Forecasting
Date/Time: November 21, 2018 / 13:40 - 14:30
Place: FENS L056
Abstract: In this talk, I will focus on developing efficient Machine Learning algorithms for two situations. One situation is high dimensional, small sampled and noisy data situations as in neuroscience, biology or geology where data collection is expensive. This phenomenon is known as curse of dimensionality that causes overfitting which is often an obstacle for using machine learning techniques. One approach to mitigate overfitting is a stochastic regularizer – dropout that is easier to implement but it does not take the structure of the data into account. We formulated a structured stochastic regularization that relies on feature grouping for a high dimensional, scarce data which suffers from overfitting. Using a fast clustering algorithm, we define a family of groups of features that capture feature covariations. Inside a stochastic gradient descent loop, we then randomly average these features. Another challenging situation would be updating a machine learning model with streaming data without iterating through previously seen data. This is also known as “online learning” and one application is forecasting time series. The performance of online learning algorithms is evaluated by the regret. Inspired by idea of calibration, we introduce a new local regret definition as an average of gradients of losses computed using the corresponding forecasts. I will discuss the properties of this new definition of regret.
Bio: Sergul has been an Assistant Professor at the department of Electrical and Computer Engineering of Stevens Insitute of Technology (SIT) in August 2018. Before joining SIT, Sergul was a Machine Learning Scientist at Amazon’s demand forecasting where she built neural network models to predict demands of millions of products to enable better in-stock positions. She is also an associate member of Parietal team at Inria, Saclay, France where she works on novel regularization methods for machine learning. She received her PhD degree from the Signal and Image Processing Institute at University of Southern California in 2014. Her PhD work was on developing robust connectivity measures for neuroimaging data. Prior to Amazon, Sergul was a postdoctoral researcher at Columbia University where she implemented machine learning models for EEG data. She then spent a year as a Data Scientist at JP Morgan. She received her B.S. and M.S. degrees in Electrical and Electronics Engineering from Bogazici University, Istanbul where she developed machine learning techniques to investigate biomedical signals. She was a recipient of the Viterbi School of Engineering Doctoral fellowship and was recognized as a 2014 USC Ming Hsieh Institute Ph.D. Scholar.
Contact: Semih Onur Sezer & Öznur Taştan& Erdinç Öztürk & Emre Özlü